Tutorials
There will be three tutorials at CAMSAP 2023:
Tutorial 1: A Short Introduction to Canonical Correlation Analysis by
Bio:
N. Sidiropoulos is the Louis T. Rader Professor of Electrical and Computer Engineering at the University of Virginia. He earned his Ph.D. in Electrical Engineering from the University of Maryland–College Park, in 1992. He has served on the faculty of the University of Minnesota, and the Technical University of Crete, Greece. His research interests are in signal processing, communications, optimization, tensor decomposition, and factor analysis, with applications in machine learning and communications. He received the NSF/CAREER award in 1998, the IEEE Signal Processing Society (SPS) Best Paper Award in 2001, 2007, 2011, and 2023, and his students received four IEEE SPS conference best paper awards. Sidiropoulos has authored a Google Classic Paper in Signal Processing (on multicast beamforming), and his tutorial on tensor decomposition is ranked #1 in Google Scholar metrics for IEEE Transactions in Signal Processing (TSP) and tops the charts of the most popular / most frequently accessed TSP papers in IEEExplore. He served as IEEE SPS Distinguished Lecturer (2008-2009), Vice President of IEEE SPS (2017-2019), and as chair of the IEEE Fellow evaluation committee of SPS (2020-2021). He received the 2010 IEEE Signal Processing Society Meritorious Service Award, and the 2013 Distinguished Alumni Award from the ECE Department of the University of Maryland. He is a Fellow of IEEE (2009) and a Fellow of EURASIP (2014). He received the EURASIP Technical Achievement Award in 2022, and the IEEE SPS Claude Shannon - Harry Nyquist Technical Achievement Award in 2023.
Paris A. Karakasis (Graduate Student Member, IEEE) received the Diploma and M.Sc. degrees in electrical and computer engineering from the Technical University of Crete, Chania, Greece, in 2017 and 2019, respectively. He is currently pursuing the Ph.D. degree with the Electrical and Computer Engineering Department, University of Virginia, Charlottesville, VA, USA. His research interests include signal processing, numerical optimization, machine learning, tensor decomposition, and graph mining. His work includes multiple contributions and publications on the theory and practice of Canonical Correlation Analysis, with applications in fMRI imaging, graph mining, and wireless communications.
Paris A. Karakasis (Graduate Student Member, IEEE) received the Diploma and M.Sc. degrees in electrical and computer engineering from the Technical University of Crete, Chania, Greece, in 2017 and 2019, respectively. He is currently pursuing the Ph.D. degree with the Electrical and Computer Engineering Department, University of Virginia, Charlottesville, VA, USA. His research interests include signal processing, numerical optimization, machine learning, tensor decomposition, and graph mining. His work includes multiple contributions and publications on the theory and practice of Canonical Correlation Analysis, with applications in fMRI imaging, graph mining, and wireless communications.
Abstract: Oftentimes, we have the ability to observe and jointly process different data sources/modalities, like text, sound, image, and video. The motivation behind handling them jointly is multifold as data fusion enables understanding associations and dependencies across different data sources, but also improving our estimation capabilities under adverse conditions (e.g., in the presence of strong noise or inferences). In many applications, the task of interest is to detect and estimate common latent factors indirectly, based on the observed modalities (a.k.a. ``views''). As we will discuss, this task can be tackled using Canonical Correlation Analysis (CCA), a powerful multivariate statistical method that aims to uncover latent relationships between two (or more) data sources. In this tutorial, we will explore the fundamental concepts and principles of CCA, its underlying assumptions, and the step-by-step process of performing CCA. We will also discuss the interpretation of CCA via a generative model, various CCA problem formulations in the linear and the nonlinear (``deep'') regime, as well as its practical applications in multimodal learning, graph mining, communications, and biomedical signal processing.
Tutorial 2: Integrated Sensing and Communications with Reconfigurable Intelligent Surfaces by
Bio: TBA
Abstract: Integrated sensing and communications (ISAC) are envisioned to be an integral part of future wireless systems, especially when operating at the millimeter-wave (mmWave) and terahertz (THz) frequency bands. Operating at these high frequencies is challenging due to the penetrating pathloss, which is so severe that the non-line-of-sight paths can be too weak to be of any practical use, preventing reliable communication or sensing. Recent years have witnessed a growing research and industrial attention in using reconfigurable intelligent surfaces (RISs) to modify the harsh propagation environment and establish reliable links for communication in Multiple-Input Multiple-Output (MIMO) systems. However, unlike the comprehensive treatment that RISs have been receiving in the context of their usage to empower wireless communications, a systematic presentation of their application to sensing as well as ISAC, along with their associated signal processing challenges, have not yet been provided. In this tutorial, we will provide an overview of the application and potential benefits of RISs for sensing and ISAC systems, aiming to encapsulate and highlight the potential benefits and main signal processing challenges which arise from such applications of this emerging technology. Our goal is to expose the existing explored directions and exciting research opportunities arising from the usage of RISs, which have already vastly impacted the wireless communications community, for sensing systems, traditionally studied by signal processing researchers and practitioners.
Tutorial 3: Hearables: Real World Applications of Interpretable AI by
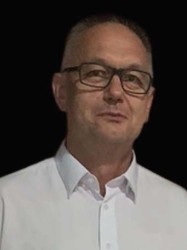
Bio: Danilo P. Mandic is a Professor of Machine Intelligence with Imperial College London, UK, and has been working in the areas of machine intelligence, statistical signal processing, big data, data analytics on graphs, bioengineering, and financial modelling. He is a Fellow of the IEEE and the current President of the International Neural Networks Society (INNS). Dr Mandic is a Director of the Financial Machine Intelligence Lab at Imperial and has more than 600 publications in international journals and conferences. Dr Mandic is a 2019 recipient of the Dennis Gabor Award for "Outstanding Achievements in Neural Engineering", given by the International Neural Networks Society. He was a 2018 winner of the Best Paper Award in IEEE Signal Processing Magazine for his article on tensor decompositions for signal processing applications, and a 2021 winner of the Outstanding Paper Award in the International Conference on Acoustics, Speech and Signal Processing (ICASSP) series of conferences. He has given about 70 Keynote and Tutorial lectures in international conferences and was appointed by the World University Service
(WUS), as a Visiting Lecturer within the Brain Gain Program (BGP), in 2015. Dr Mandic is a 2014 recipient of President Award for Excellence in Postgraduate Supervision at Imperial College and holds several patents on Hearables.
Harry J. Davies is a Meta Research Fellow with Imperial College London, specialising in bio-signal processing and interpretable AI with application to Hearables. He pioneered domain-aware Data Augmentation for wearables and received the Editor’s choice award for his paper “In-Ear SpO2: A Tool for Wearable, Unobtrusive Monitoring of Core Blood Oxygen Saturation”. He was also the first to demonstrate that wearable photoplethysmography (PPG) can be used to screen for chronic obstructive pulmonary disease (COPD), resulting in a patent. Dr Davies has published in numerous journals and conferences at the intersection of biomedical engineering, signal processing, and health technology. He was invited to join several of the world’s foremost photoplethysmography researchers to co-author “The 2023 Wearable Photoplethysmography Roadmap” on respiratory monitoring from In-Ear PPG. He has attracted funding from Sony Corporation and Meta and is currently working on cognitive state estimation in virtual reality environments.
Harry J. Davies is a Meta Research Fellow with Imperial College London, specialising in bio-signal processing and interpretable AI with application to Hearables. He pioneered domain-aware Data Augmentation for wearables and received the Editor’s choice award for his paper “In-Ear SpO2: A Tool for Wearable, Unobtrusive Monitoring of Core Blood Oxygen Saturation”. He was also the first to demonstrate that wearable photoplethysmography (PPG) can be used to screen for chronic obstructive pulmonary disease (COPD), resulting in a patent. Dr Davies has published in numerous journals and conferences at the intersection of biomedical engineering, signal processing, and health technology. He was invited to join several of the world’s foremost photoplethysmography researchers to co-author “The 2023 Wearable Photoplethysmography Roadmap” on respiratory monitoring from In-Ear PPG. He has attracted funding from Sony Corporation and Meta and is currently working on cognitive state estimation in virtual reality environments.
Abstract: The Hearables paradigm, that is, in-ear sensing of neural function and vital signs, is an emerging solution for 24/7 discrete health monitoring. The tutorial starts by introducing our own Hearables device, which is based on an earplug with the embedded electrodes, optical, acoustic, mechanical and temperature sensors. We show how such a miniaturised embedded system can be can used to reliably measure the Electroencephalogram (EEG), Electrocardiogram (ECG), pulse, respiration, temperature, blood oxygen levels, and behavioural cues. Unlike standard wearables, such an inconspicuous Hearables earpiece benefits from the relatively stable position of the ear canal with respect to vital organs to operate robustly during daily activities. However, this comes at a cost of weaker signal levels and exposure to noise. This opens novel avenues of research in Machine Intelligence for eHealth, with both numerous challenges and opportunities for algorithmic solutions. We describe how our hearables sensor can be used, inter alia, for automatic sleep monitoring and screening of chronic obstructive pulmonary disease. For Hearables to provide a paradigm shift in eHealth, they require domain-aware Machine Intelligence, to detect, estimate, and classify the notoriously weak physiological signals from the ear-canal. To this end, the second part of our tutorial is focused on interpretable AI. This is achieved by using first principles to explain the principles of convolutional neural networks (CNNs) through matched filters. This allows us to revisit the operation of CNNs and show that their key component – the convolutional layer – effectively performs matched filtering of its inputs with a set of templates (filters, kernels) of interest. This serves as a vehicle to establish a compact and physically meaningful perspective of the whole convolution-activation-pooling chain, which allows for a theoretically well founded and physically meaningful insight into the overall operation of CNNs. This is shown to help mitigate their interpretability and explainability issues, together with providing intuition for further developments and novel physically meaningful ways of their initialisation. We demonstrate this effect in the context of Hearables, specifically the Ear-ECG, through a convolutional neural network designed for R-peak detection. We show that fully interpretable networks such as these are pivotal in the integration of AI into medicine, as they dispel the black box nature of deep learning and allow clinicians to make informed decisions based off network outputs. Owing to their unique Collocated Sensing nature, Hearables record a rich admixture of information from several physiological variables, motion, muscle artefacts and noise. For example, even a standard EEG measurement contains a weak ECG and muscle artefacts, which are typically treated as bad data and are subsequently discarded. In the quest to exploit all the available information (no data is bad data), the final section of the tutorial focuses on a novel class of encoder-decoder networks which, taking the advantage from the collocation of information, maximise data utility. We focus on our own Correncoder architecture and demonstrate its ability to learn a shared latent space between the model input and output, making it a deep-NN generalisation of partial least squares (PLS). Finally, real-world applications of the Correncoder are presented, ranging from transforming Photoplethysmography (PPG) into respiratory signals, through to making sense from artefacts and decoding implanted brain electrical signals into movement.